Sim-to-Lab-to-Real: Safe Reinforcement Learning with Shielding and Generalization Guarantees
Published in Artificial Intelligence, 2022
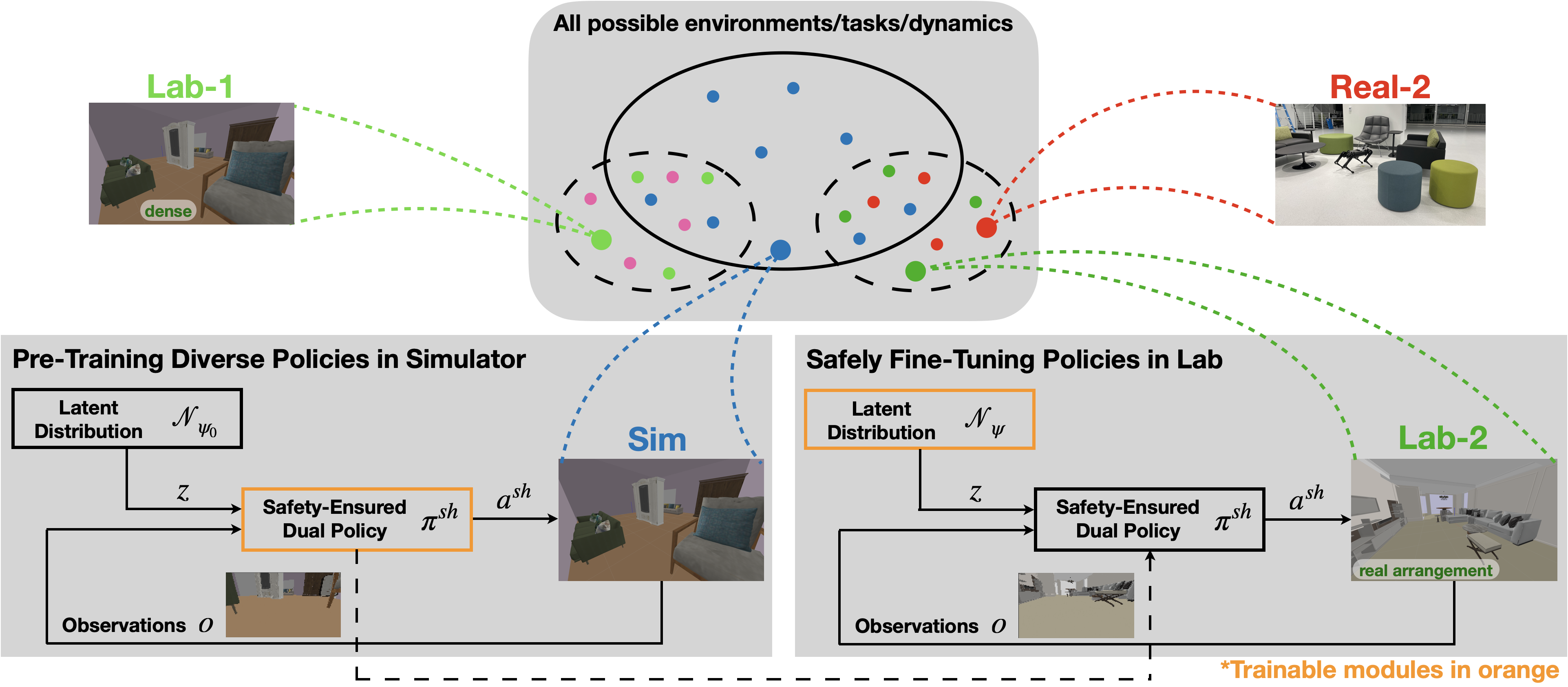
We leverage a middle-level training stage, Lab, between Sim and Real to safely close the Sim-to-Real gap in ego-vision indoor navigation task. Compared to Sim training, Lab training is (1) more realistic and (2) more safety-critical.
For safe Sim-to-Lab transfer, we learn a safety critic with Hamilton-Jacobi reachability-based RL and apply a supervisory control scheme to shield unsafe actions during exploration.
For safe Lab-to-Real transfer, we use the Probably Approximately Correct (PAC)-Bayes Control framework to provide lower bounds (70-90%) on the expected performance and safety of policies in unseen environments.